Chen
Tessler
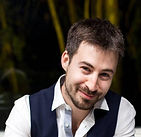
Chen Tessler - P.hD Candidate in Reinforcement Learning
I'm currently pursuing my P.hD in Reinforcement Learning at the Technion Institute of Technology, Israel, under the supervision of Prof. Shie Mannor.
Reinforcement Learning (RL) is a learning paradigm which highly resembles how we, as humans, learn. The agent, i.e., the learning algorithm, learns through interaction with the environment. By observing the current state of the system, it decides which action to take, after which the environment transitions to a new state and produces the agent with a reward. The goal of the agent is to maximize the accumulative reward.
In my research I focus on Deep Reinforcement Learning (DRL), in which we use Deep Learning techniques (neural networks) to solve reinforcement learning problems.
Specifically, I am interested in finding the problems which are unique to DRL (e.g., which occur due to non-linear function approximation) and how they can be solved / mitigated in order to improve empirical performance.
Education
2019 -
P.hD. Candidate - Reinforcement Learning, Technion
2017 - 2019
M.Sc. - Reinforcement Learning, Technion
2014 - 2017
B.Sc. - Electrical Engineering, Technion
2009 - 2012
B.A. - IT Management, Ben Gurion University
Experience
2017
Machine Learning Research Intern, Google
2015 - 2017
Performance Verification, Chip Design, Mellanox
Publications
Policy gradient methods suffer from large variance and instability during training. In this work, we propose a conservative update rule for off-policy RL intended to overcome these difficulties.
Stabilizing Off-Policy Reinforcement Learning with Conservative Policy Gradients
Chen Tessler, Nadav Merlis and Shie Mannor
arXiv
We propose a method for learning distributional policies, policies which are not limited to parametric distribution functions (e.g., Gaussian and Delta). This approach overcomes sub-optimal local extremum in continuous control regimes.
Distributional Policy Optimization: An Alternative Approach for Continuous Control
Chen Tessler*, Guy Tennenholtz* and Shie Mannor
NeurIPS 2019
Action Robust is a special case of robustness, in which the agent is robust to uncertainty in the performed action. We show (theoretically) that this form of robustness has efficient solutions and (empirically) results in policies which are robust to common uncertainties in robotic domains.
Action Robust Reinforcement Learning and Applications in Continuous Control
Chen Tessler*, Yonathan Efroni* and Shie Mannor
ICML 2019
Learning a policy which adheres to behavioral constraints is an important task. Our algorithm, RCPO, enables the satisfaction of not only discounted constraints but also average and probabilistic, in an efficient manner.
Reward Constrained Policy Optimization
Chen Tessler, Daniel J. Mankowitz and Shie Mannor
ICLR 2019
We propose a lifelong learning system that has the ability to reuse and transfer knowledge from one task to another while efficiently retaining the previously learned knowledge-base. Knowledge is transferred by learning reusable skills to solve tasks in Minecraft, a popular video game which is an unsolved and high-dimensional lifelong learning problem.
A Deep Hierarchical Approach to Lifelong Learning in Minecraft
Chen Tessler*, Shahar Givony*, Tom Zahavy*, Daniel J. Mankowitz* and Shie Mannor
AAAI 2017
Awards
2017
Meyer foundation fellowship for graduate studies, Technion
2017
EMET Excellence program graduate, Technion
2017
1st place - Yehoraz Kasher project contest, Technion
2016
1st place - David Cohn Corporate Entrepreneurship Award, Technion